You will learn to:
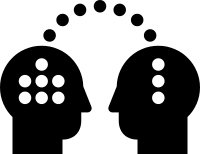
- use
dplyr
/purrr
for efficient data manipulation - tidying linear models using
broom
- managing workflow by keeping related things together in one
tibble
.
4 May 2017
dplyr
/ purrr
for efficient data manipulationbroom
tibble
.Tutorial based on the great conference by Hadley Wickham
progress bar will be added
gapminder
library("gapminder") gapminder %>% ggplot(aes(x = year, y = lifeExp, group = country)) + geom_line()
by_country <- gapminder %>% mutate(year1950 = year - 1950) %>% group_by(continent, country) %>% nest() by_country
# A tibble: 142 x 3 continent country data <fctr> <fctr> <list> 1 Asia Afghanistan <tibble [12 x 5]> 2 Europe Albania <tibble [12 x 5]> 3 Africa Algeria <tibble [12 x 5]> 4 Africa Angola <tibble [12 x 5]> 5 Americas Argentina <tibble [12 x 5]> 6 Oceania Australia <tibble [12 x 5]> 7 Europe Austria <tibble [12 x 5]> 8 Asia Bahrain <tibble [12 x 5]> 9 Asia Bangladesh <tibble [12 x 5]> 10 Europe Belgium <tibble [12 x 5]> # ... with 132 more rows
year1950
will help to get count oldest datecontinent
to group_by()
to keep the infogapminder %>% filter(country == "Germany") %>% select(-country, -continent)
# A tibble: 12 x 4 year lifeExp pop gdpPercap <int> <dbl> <int> <dbl> 1 1952 67.500 69145952 7144.114 2 1957 69.100 71019069 10187.827 3 1962 70.300 73739117 12902.463 4 1967 70.800 76368453 14745.626 5 1972 71.000 78717088 18016.180 6 1977 72.500 78160773 20512.921 7 1982 73.800 78335266 22031.533 8 1987 74.847 77718298 24639.186 9 1992 76.070 80597764 26505.303 10 1997 77.340 82011073 27788.884 11 2002 78.670 82350671 30035.802 12 2007 79.406 82400996 32170.374
by_country %>% filter(country == "Germany") %>% pull(data) # dplyr 0.6, .$data for dplyr 0.5
[[1]] # A tibble: 12 x 5 year lifeExp pop gdpPercap year1950 <int> <dbl> <int> <dbl> <dbl> 1 1952 67.500 69145952 7144.114 2 2 1957 69.100 71019069 10187.827 7 3 1962 70.300 73739117 12902.463 12 4 1967 70.800 76368453 14745.626 17 5 1972 71.000 78717088 18016.180 22 6 1977 72.500 78160773 20512.921 27 7 1982 73.800 78335266 22031.533 32 8 1987 74.847 77718298 24639.186 37 9 1992 76.070 80597764 26505.303 42 10 1997 77.340 82011073 27788.884 47 11 2002 78.670 82350671 30035.802 52 12 2007 79.406 82400996 32170.374 57
by_country_lm <- by_country %>% mutate(model = map(data, ~ lm(lifeExp ~ year1950, data = .x))) by_country_lm
# A tibble: 142 x 4 continent country data model <fctr> <fctr> <list> <list> 1 Asia Afghanistan <tibble [12 x 5]> <S3: lm> 2 Europe Albania <tibble [12 x 5]> <S3: lm> 3 Africa Algeria <tibble [12 x 5]> <S3: lm> 4 Africa Angola <tibble [12 x 5]> <S3: lm> 5 Americas Argentina <tibble [12 x 5]> <S3: lm> 6 Oceania Australia <tibble [12 x 5]> <S3: lm> 7 Europe Austria <tibble [12 x 5]> <S3: lm> 8 Asia Bahrain <tibble [12 x 5]> <S3: lm> 9 Asia Bangladesh <tibble [12 x 5]> <S3: lm> 10 Europe Belgium <tibble [12 x 5]> <S3: lm> # ... with 132 more rows
library("broom") models <- by_country_lm %>% mutate(glance = map(model, glance), rsq = glance %>% map_dbl("r.squared"), tidy = map(model, tidy), augment = map(model, augment)) models
# A tibble: 142 x 8 continent country data model glance <fctr> <fctr> <list> <list> <list> 1 Asia Afghanistan <tibble [12 x 5]> <S3: lm> <data.frame [1 x 11]> 2 Europe Albania <tibble [12 x 5]> <S3: lm> <data.frame [1 x 11]> 3 Africa Algeria <tibble [12 x 5]> <S3: lm> <data.frame [1 x 11]> 4 Africa Angola <tibble [12 x 5]> <S3: lm> <data.frame [1 x 11]> 5 Americas Argentina <tibble [12 x 5]> <S3: lm> <data.frame [1 x 11]> 6 Oceania Australia <tibble [12 x 5]> <S3: lm> <data.frame [1 x 11]> 7 Europe Austria <tibble [12 x 5]> <S3: lm> <data.frame [1 x 11]> 8 Asia Bahrain <tibble [12 x 5]> <S3: lm> <data.frame [1 x 11]> 9 Asia Bangladesh <tibble [12 x 5]> <S3: lm> <data.frame [1 x 11]> 10 Europe Belgium <tibble [12 x 5]> <S3: lm> <data.frame [1 x 11]> # ... with 132 more rows, and 3 more variables: rsq <dbl>, tidy <list>, # augment <list>
models %>% ggplot(aes(x = rsq, y = reorder(country, rsq))) + geom_point(aes(colour = continent)) + theme(axis.text.y = element_text(size = 6))
models %>% filter(rsq < 0.55) %>% unnest(data) %>% ggplot(aes(x = year, y = lifeExp)) + geom_line(aes(colour = continent)) + facet_wrap(~ country) + theme(legend.justification = c(1, 0), legend.position = c(1, 0))
models %>% unnest(tidy) %>% select(continent, country, rsq, term, estimate) %>% spread(term, estimate) %>% ggplot(aes(x = `(Intercept)`, y = year1950)) + geom_point(aes(colour = continent, size = rsq)) + geom_smooth(se = FALSE, method = "loess") + scale_size_area() + labs(x = "Life expectancy (1950)", y = "Yearly improvement")
library(gganimate) gapminder %>% ggplot(aes(x = gdpPercap, y = lifeExp, size = pop, color = continent, frame = year)) + geom_point() + scale_x_log10() -> p gganimate(p, 'img/09_gapminder.gif')